How does generative AI work
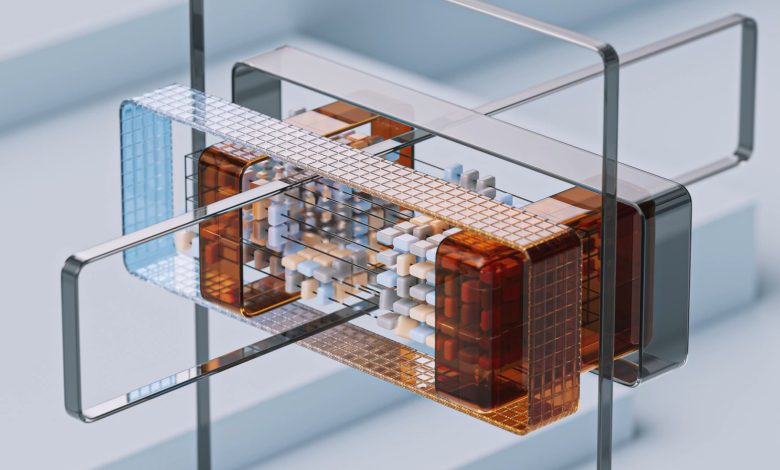
Have you ever wondered how a computer can create music, art, or even generate entire pieces of writing? The answer lies in the fascinating world of generative artificial intelligence (AI). From composing symphonies to generating human-like conversations, generative AI has been making waves in various industries and capturing the imagination of people around the globe. In this article, we will delve into the inner workings of generative AI and explore how it harnesses the power of machine learning and neural networks to produce content that is eerily human-like.
What is Generative AI?
Generative AI, also known as generative adversarial networks (GANs), is a fascinating area of artificial intelligence that focuses on creating new and original content. Unlike traditional AI algorithms that are designed to recognize patterns or make decisions based on data, generative AI is capable of producing completely novel outputs such as images, music, and even text. One of the key components of generative AI is its ability to learn from examples and then generate new content that closely resembles the input data.
What sets generative AI apart from other forms of AI is its creative potential. By leveraging complex neural network architectures and advanced algorithms, it can produce content that mimics human creativity. This technology has a wide range of applications, including art generation, content creation for marketing and advertising, and even drug discovery in the pharmaceutical industry. As the capabilities of generative AI continue to expand, it opens up exciting possibilities for innovation across various industries.
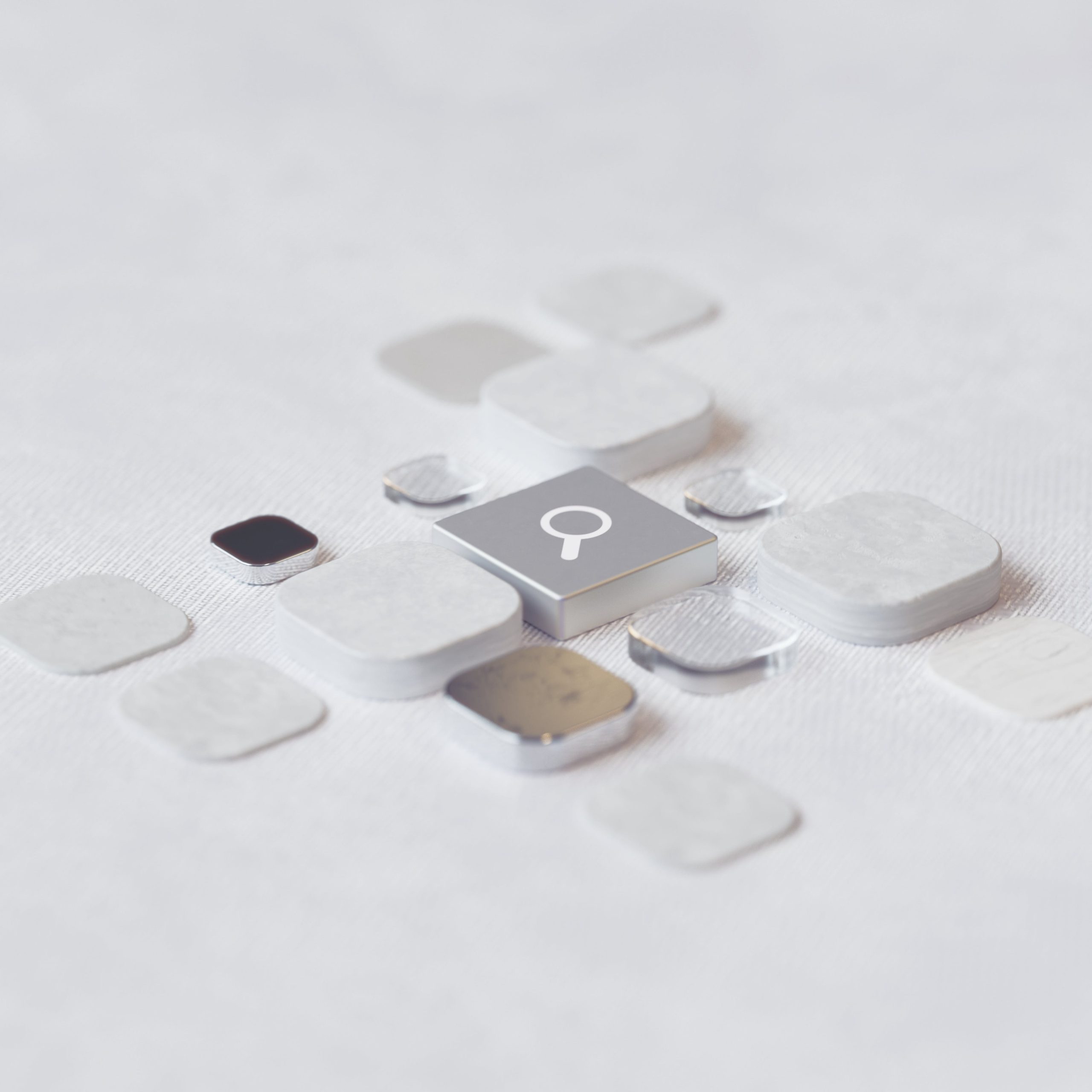
How Does Generative AI Learn?
Generative AI, such as GANs (Generative Adversarial Networks), learns through a process of competition and cooperation. In a GAN system, two neural networks – the generator and the discriminator – engage in a continual game of one-upmanship. The generator creates synthetic data, while the discriminator attempts to distinguish between real and fake examples. Through this adversarial process, both networks learn from each other’s successes and failures, ultimately driving improvement in the generation of realistic outputs.
Furthermore, generative AI also learns through a training process that involves exposure to vast amounts of diverse data. This immersion allows the AI to identify patterns, correlations, and dependencies within the information it encounters. By analyzing this data, generative AI can then create new content that reflects its understanding of these underlying structures. Consequently, as generative AI continues to evolve and refine its capabilities through learning from a wide variety of inputs, it becomes increasingly adept at generating nuanced and sophisticated outputs.
Applications of Generative AI
Generative AI technology has revolutionized various industries, offering unprecedented opportunities for innovation and creativity. One prominent application lies in the field of art and design, where it can generate unique and visually stunning creations. From paintings to digital graphics, generative AI has been instrumental in pushing the boundaries of expression and providing artists with new tools to explore their craft. Moreover, in the realm of content creation, there is immense potential for generating realistic images, videos, and audio that can enhance virtual experiences and storytelling.
Additionally, generative AI has made significant strides in the medical field by aiding in drug discovery and development. Through its ability to simulate molecular structures and predict potential drug candidates, generative AI has accelerated research processes that would have traditionally taken years to accomplish. This application not only holds promise for advancing pharmaceutical innovation but also stands as a testament to the profound impact generative AI can have on humanity’s well-being. Furthermore, the technology’s use in creating synthetic data for training medical imaging algorithms showcases its potential to improve healthcare diagnostics and treatment planning.
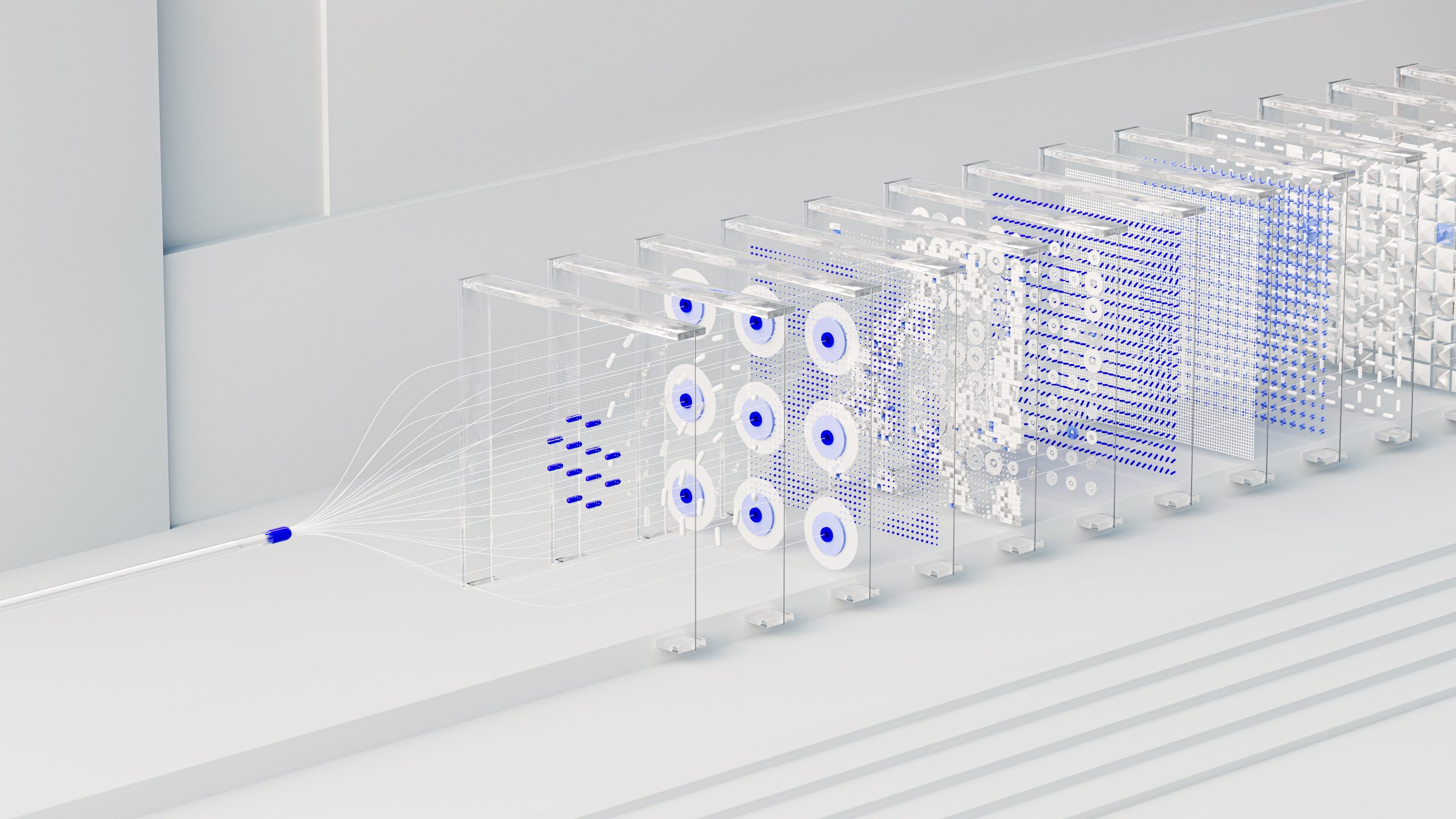
Challenges and Limitations
While generative AI holds immense potential for revolutionizing various industries, it also comes with its fair share of challenges and limitations. One significant challenge is the ethical implications surrounding the use of generated content, particularly in areas like artwork, music, and writing. The issue of ownership, copyright infringement, and the blurring lines between original and AI-generated work raises complex legal and moral questions that need to be addressed.
Moreover, the limitations of current generative AI models often manifest in their inability to understand context or produce truly human-like creativity. This means that while they can generate impressive outputs based on existing data patterns, they may struggle with originality or grasping nuanced human emotions. Additionally, lack of diversity in training data could lead to biased outputs, posing a risk to inclusivity and fairness when applied in real-world scenarios. Overcoming these challenges will require not only advancements in technology but also thoughtful regulations and proactive measures to mitigate potential risks.
In conclusion, as we delve deeper into the capabilities of generative AI technology, it’s crucial to acknowledge these challenges and actively work towards mitigating their impact. Embracing this transformative tool while addressing its ethical considerations will ultimately shape a future where generative AI serves as a force for positive innovation rather than a source of contention.
Ethical Considerations
Ethical considerations surrounding generative AI are crucial in the current technological landscape. As this technology becomes increasingly advanced, questions about the ethical use and implications of generative AI arise. One key concern is the potential for misuse, such as creating deepfake content or manipulating information for malicious purposes. Additionally, there are concerns about bias in data used to train these systems, which could lead to unfair or discriminatory outcomes. Transparency and accountability in AI development are essential to address these issues and ensure that generative AI is deployed ethically.
Moreover, the impact of generative AI on job displacement and labor markets cannot be ignored. While it may streamline certain processes, its widespread adoption could lead to significant disruptions in various industries, raising questions about the socioeconomic implications of this technology. Furthermore, privacy concerns related to the generation of synthetic data and the potential misuse of personal information are also valid points for ethical consideration. Addressing these ethical considerations will require collaboration between technologists, ethicists, policymakers, and other stakeholders to establish regulations and guidelines that uphold ethical standards while harnessing the benefits of generative AI for society’s advancement.
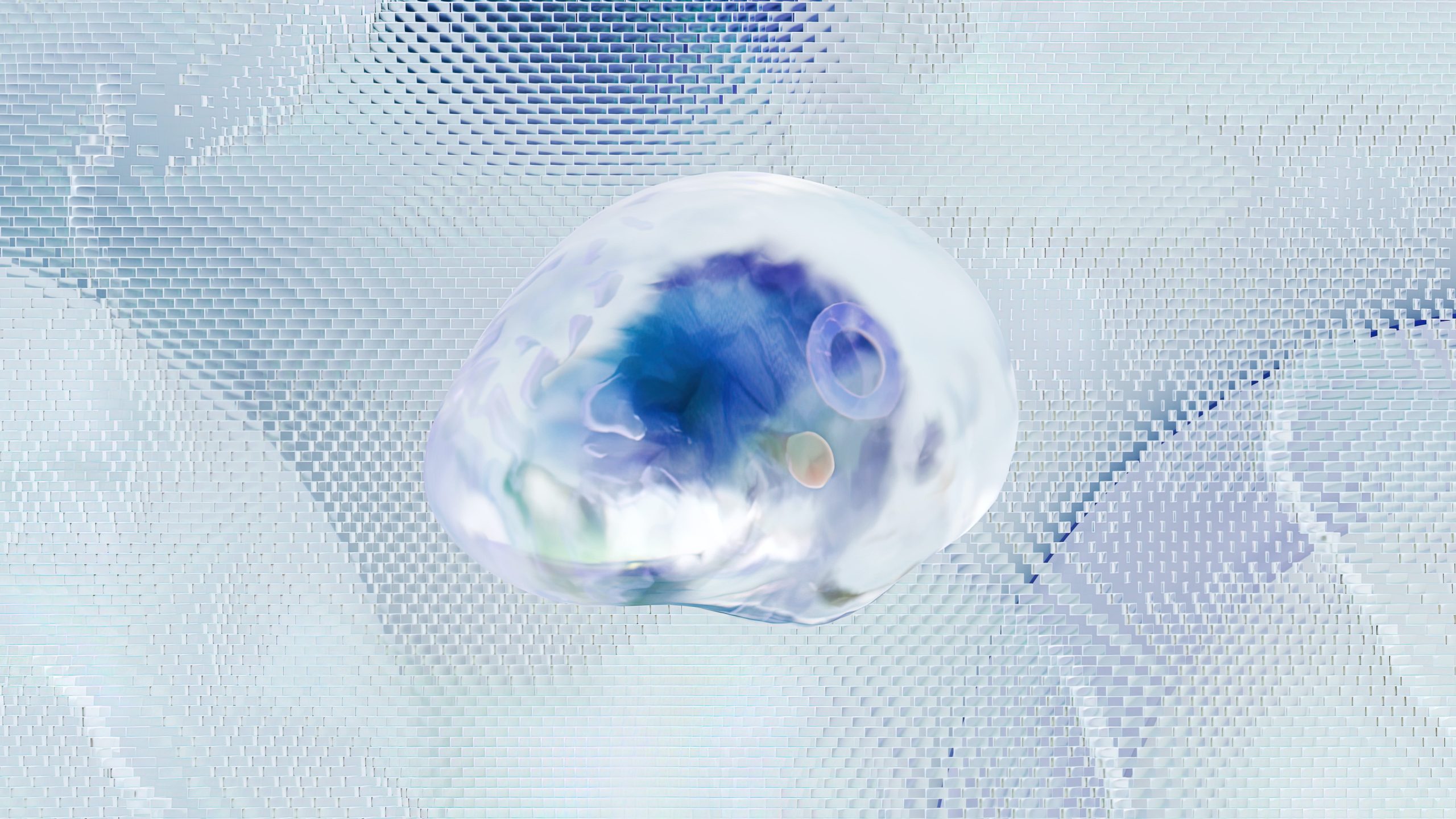
Conclusion: The Future of Generative AI
As we delve into the future of generative AI, it’s important to acknowledge the potential impact and ethical considerations surrounding its development. While generative AI holds promise in revolutionizing various industries such as art, music, and writing, there are also concerns about its misuse for creating fake content or misinformation. As technology advances, it will be crucial for policymakers and developers to work together to establish clear guidelines and regulations to ensure responsible use of generative AI.
Moreover, the future of generative AI also presents an exciting opportunity for collaboration between humans and machines. Rather than fearing the obsolescence of human creativity, we can anticipate a future where generative AI acts as a tool to enhance human artistic expression and problem-solving abilities. This symbiotic relationship has the potential to unlock new levels of innovation and creativity that were previously unimaginable. As we move forward, it’s essential to embrace this co-creative potential while addressing any ethical challenges that may arise along the way.